No one can deny AI has gained momentum in the music industry last year. Just like autotune sparked debate a few years back, then music NFTs and web3 in music were all over the news in the industry outlets and communities, AI is currently a new agenda that every artist, fan, and rights-holder has surely heard of.
But it's not just generative text-to-music AI tools or voice cloning that is in place, albeit they take centre stage in the copyright, ethics, and production debate. There are plenty of other artificial intelligence-powered tools that are rarely spoken of for all kinds of workflows—from sound synthesis and stem separation through sampling to mixing and mastering.
To talk about AI in music and where we're heading in this regard, we sat down with Max Hilsdorf, data scientist and musicologist—Max studied both musicology and data science in university, which gave him a solid foundation for working in this interdisciplinary field. He has worked extensively on automated music tagging in his thesis as well as at Cyanite, a German music analysis startup.
Today, he has shifted more towards AI education—as a Data Science Consultant at statworx, he holds workshops on data science & AI across various industries and sharing his knowledge about music AI on his Medium blog.
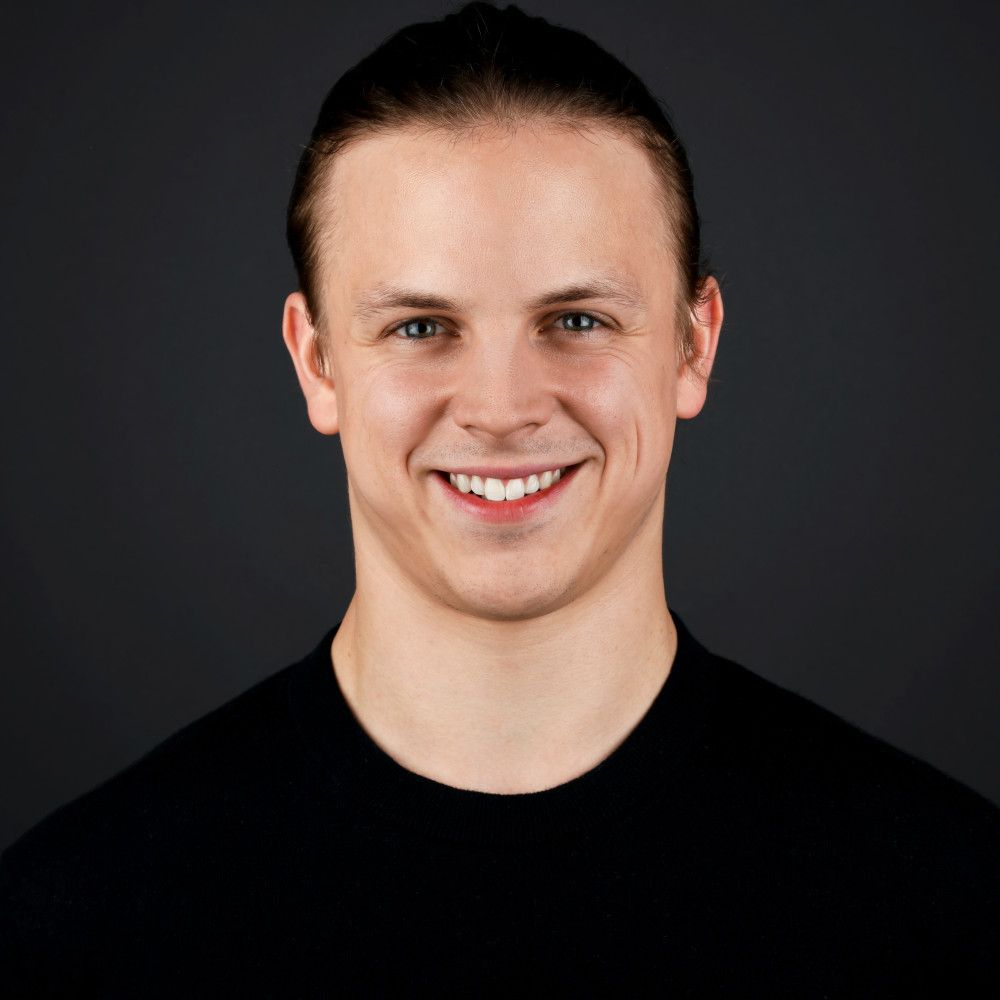
How AI is used to create or analyse music—even musicologists cannot answer this with certainty.
Traditional computer programs rely on explicit rules to solve problems. For example, a song’s mood could be analysed based on its key (e.g., major → happy, minor → sad). However, the real world is more complicated than that. For example, “Happy” by Pharrell Williams is played in a minor key. But what makes this song so positive, then? The truth is that even musicologists cannot answer this question with certainty.
When we build AI systems, we bypass this problem by letting the computer extract its own decision rules. We can now simply feed it a large corpus of music data and have it learn the relevant patterns autonomously. This method is called machine learning. With generative music, it is the same process, fundamentally. Only here is the AI incentivised to replicate musical patterns, not just understand them.
When it comes to AI's understanding and replicating different musical styles and genres, due to the complex nature of large machine learning models, we do not know what is going on under the hood, precisely. The only thing we can do is infer general statements from the way the AI was trained and from the dataset used for that. Simply put, an AI starts with zero musical knowledge. In an iterative process, the training phase, they learn musical patterns from their training material and try to create music that is almost indistinguishable from the real tracks. With a large enough dataset, you can run this training process over and over again until the results are acceptable.
The kind and quality of an AI’s output depends first and foremost on the data used to train it. If you train an AI solely on country music, it will also generate country music. But ChatGPT cannot only write poems; it can also come up with birthday cards, right? That is why AI models like Google’s MusicLM or Meta’s MusicGen are trained on music from various styles. This way, they can serve as general-purpose models for music creation. In the future, we might be able to generate music just like we can generate texts today.
Acquiring high-quality data is a massive challenge, and doing so ethically seems almost impossible.
The two biggest problems are a lack of computing resources and high-quality data. GPT-3, the predecessor to ChatGPT, was trained on more than 75TB of text data. This equates to roughly 5 million hours of music or 100 million songs. Acquiring high-quality data on that scale is a massive challenge, and doing so ethically seems almost impossible. And even if you have acquired the data, you need a huge fleet of high-end computers running in parallel for weeks. Only a few players in the market can afford this. Computing costs are likely to decrease every year, which will lower the barrier to entry to some extent. However, obtaining large high-quality music datasets in an ethical [way] will remain challenging.
Ethically, I find it hard to accept that musicians’ tracks are used to train AI systems that become direct competitors to them—all without their consent. In the past, we have seen good and bad examples. To train MusicGen, Meta relied solely on licensed tracks from stock music platforms. In my opinion, we need to strengthen the position of artists to make sure that consent and fair remuneration become the norm rather than the exception.
Copyright concerns are a problem as AI could blur the lines when it comes to authorship and intellectual property. The concept of "ethical datasets" is a critical issue here. Furthermore, AI could disrupt traditional revenue streams in the music industry. Democratization of technology inevitably leads to more players entering the market. This will urge established institutions to rethink their business model and explore new ways of creating value.
GPT-3, the predecessor to ChatGPT, was trained on more than 75TB of text data. This equates to roughly 5 million hours of music or 100 million songs.
Can machines be creative? The creative potential of randomness is often underestimated.
In my daily work as an AI educator, this must be one of the most common debates I have in my workshops: “Can machines be creative?”
I think there are two common misconceptions at play here. Firstly, human musicians are also heavily inspired by the music they have consumed in their lives. Therefore, their “training data” is reflected in all of their creative works, to some extent. This is not much different from an AI learning to reproduce patterns from its training dataset.
Secondly, the creative potential of randomness is often underestimated. If I compose piano pieces solely through dice rolls, and I do that for an infinite amount of time, I will inevitably produce every piano piece that has ever existed. If an AI takes patterns learned from the training material and recombines them with some level of randomness, novel and creative works can be achieved. Now, whether you want to call this creativity or not opens a complex philosophical debate. In practice, however, we must face the reality that AI is already producing thousands of novel pieces of art every day.
The subjective nature of music makes building music AI challenging. In principle, AI is able to see through noisy data and extract aggregated patterns that reflect the opinions of most people. I cannot stress this point enough: It all depends on the dataset. For example, if you train a mood detection AI on data from European users, the AI might not produce results that Asian people find useful. Then the question is, do we even want a universal mood detection system for everyone? When there is much subtlety and ambiguity, it might be better to build AI in a way that offers customised experiences. For example, you can already tell ChatGPT about yourself and it will adapt its answers to your background. We could have the same thing for music AI in the future.
Generative AI is an emerging field & music source separation is a keystone technology.
Speaking of the latest breakthroughs in music AI, you might not think of this at first, but music source separation is a keystone technology, in my opinion. Once we are able to reliably extract any sound or instrument from any piece of music, the possibilities are endless. Source separation will allow us to turn mono recordings into stereo, create backing tracks for any instrument, and sample any melody or sound we want. It will also enable the synthetic creation of much larger datasets, which will further boost generative music systems.
One particularly fascinating paper is called “Separate Anything You Describe” (Liu et al., 2023) and introduces an AI that can perform source separation based on a text prompt. In the future, we might be able to prompt AI to “extract the main synth in the hook, but without delay” and obtain a natural-sounding result. I'm looking forward to seeing how this technology will develop.
Generative AI is an emerging field with tremendous economic potential. I fully understand why many enter the market now to secure their slice of the pie. Time will separate the wheat from the chaff and we as consumers will be left with amazing products that make our lives better. As always, when there is hype, we tend to overestimate short-term effects and underestimate long-term effects. That is why we should not jump on the first boat we see, but keep an eye on what could create value in the long run.
Figuring out a system based on consent and fair remuneration towards music creators is a key challenge for the next years.
I predict that the quality of data will beat the quantity for most use cases, allowing for more controlled data curation.
Currently, many generative AI models are trained on large datasets crawled from the internet. This is problematic for several reasons, one being the risk of training on AI-generated material. If an AI is trained on its own outputs or those of similar models, it might reinforce its own biases instead of learning new useful patterns. You could say it becomes detached from its real, human-made training data.
While I do see this as a risk under the current paradigm, my opinion is that mindlessly crawling the entire internet and training an AI based on it is a flawed approach to begin with. Ideally, you want more control over what exactly you are feeding the model. Luckily, we have seen many cases of open-source AI models being trained on comparatively small datasets, outclassing traditional approaches. In the future, I predict that the quality of data will beat the quantity for most use cases, allowing for more selective and controlled data curation.
It is impossible to make accurate predictions for the next decade. What I can do is lay out some broad developments that I am currently seeing and that could continue in the future.
One positive impact of AI in music is its potential to democratise content creation. Many people are musically creative but have never learned to play an instrument. Generative AI can lower the barrier of entry and empower everyone to compose, produce, and share the music they love. This is clearly a positive development for society.
Obviously, one benefit of AI is also its capability to automate or speed up existing workflows. Cumbersome manual work like tagging or finding the right music for your social media post is already being enhanced or automated with AI. This gives creators and business users more time to spend on meaningful and creative tasks.
On the negative side of AI, music is just as affected by deep fakes, copyright issues, and monetisation concerns as other domains. Figuring out a system based on consent and fair remuneration towards music creators is a key challenge for the next years.
We are likely at the beginning of a technological revolution comparable to the invention of the internet or, as some would argue, the invention of electricity. For all we know, AI could lay waste on everything we know or bring unprecedented flourishing. Most likely, we will end up somewhere between these extremes, and it will be okay!